Data Science in Retail: Personalizing Customer Experiences and Optimizing Inventory
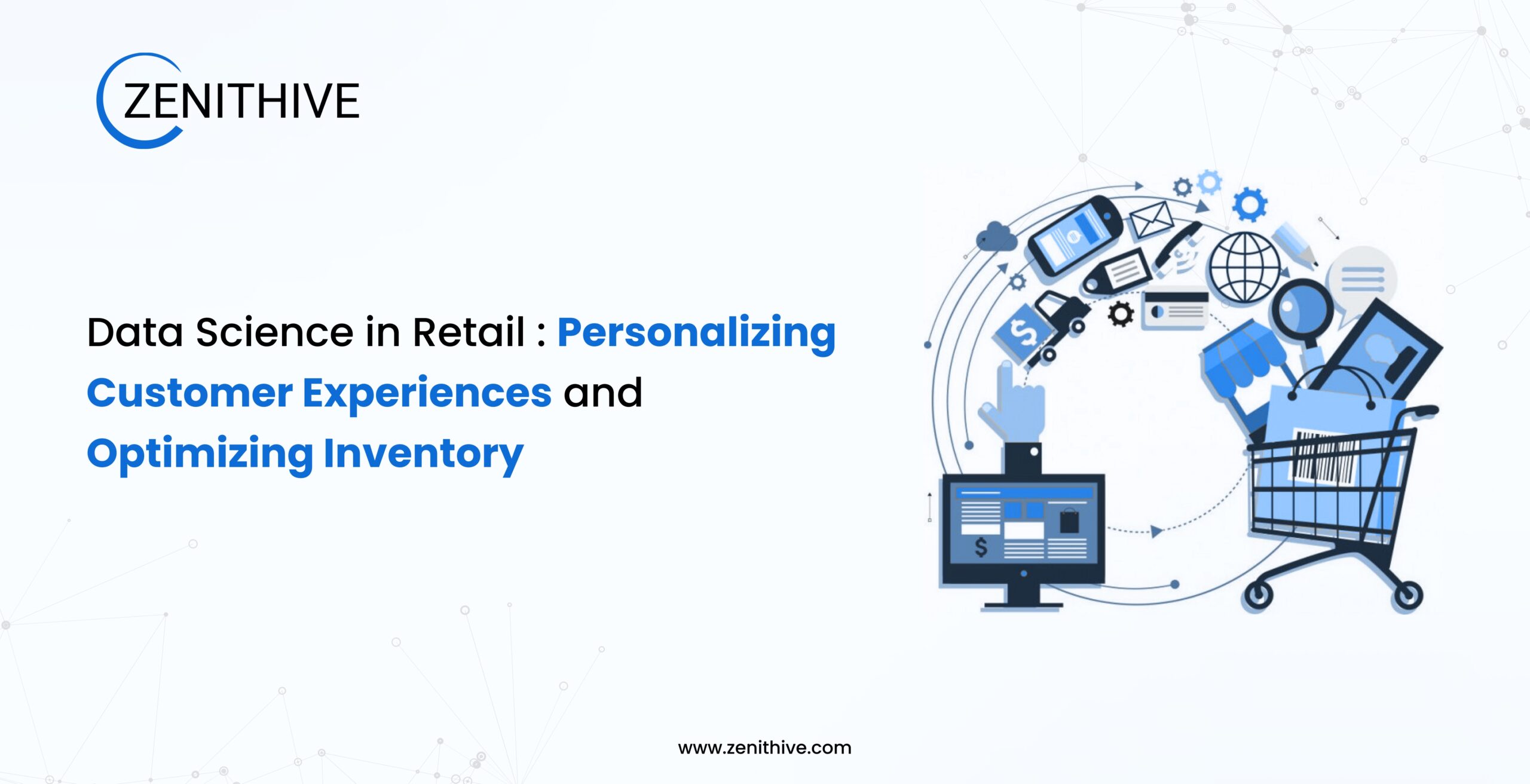
In the rapidly evolving retail world, data science has emerged as the ultimate competitive differentiator. The 2021 Inmar Intelligence Shopping Survey revealed a staggering statistic: 90% of consumers believe personalization directly influences their spending habits. This isn’t just a trend, it’s a revolutionary shift that’s redefining how retailers connect with customers, optimize inventory, and drive unprecedented growth.
The Data-Driven Retail Transformation
Decoding Customer Complexity Through Advanced Analytics
Modern retail has transcended traditional boundaries, evolving from a one-size-fits-all approach to a hyper-personalized ecosystem driven by sophisticated data analysis. Retailers now possess an unprecedented ability to synthesize multiple data streams, personal profiles, behavioral patterns, engagement metrics, and sentiment analysis, creating comprehensive customer portraits that go far beyond basic demographic information.
The complexity of contemporary consumer behavior demands equally sophisticated analytical approaches. Machine learning algorithms now parse through massive datasets, identifying subtle patterns that human analysts might overlook. For instance, a customer’s browsing history, social media interactions, and purchase history can be integrated to create a 360-degree view of their preferences, enabling retailers to anticipate needs before the customer even articulates them.
According to a study by McKinsey & Company, retailers that use advanced analytics to optimize their marketing campaigns see a 15-20% increase in marketing ROI. Additionally, a survey by Accenture found that 91% of consumers are more likely to shop with brands that recognize, remember, and provide relevant offers and recommendations.
The Personalization Imperative
Personalization has moved from a competitive advantage to a fundamental expectation. Consider these compelling statistics:
- 91% of consumers are more likely to shop with brands that recognize, remember, and provide relevant offers and recommendations (Accenture)
- Personalized email marketing delivers 6x higher transaction rates (Experian)
- Companies with advanced personalization strategies see 20% higher customer satisfaction rates (Boston Consulting Group)
AI-powered recommendation systems have become the cornerstone of this personalization revolution. Salesforce reports that retailers implementing sophisticated recommendation engines experience up to 15% higher cart conversion rates. Amazon’s recommendation engine stands as a prime example, contributing to 35% of its revenue, a demonstration of the transformative power of intelligent personalization.
Retailers are leveraging data science to deliver hyper-targeted experiences across various touchpoints. This includes personalized email campaigns, dynamic website content, real-time product recommendations, and predictive offer generation. By integrating customer data from multiple sources, retailers can create a seamless, personalized shopping experience that anticipates and fulfills customer needs.
Predictive Inventory Management: The Data Science Advantage
Traditional inventory management was reactive, responding to past trends and gut feelings. Data science has transformed this into a predictive, proactive discipline. By leveraging advanced analytics, retailers can now:
- Anticipate demand with unprecedented accuracy
- Minimize inventory carrying costs
- Reduce stockout scenarios
- Optimize supply chain efficiency
Machine learning models can now predict inventory needs by analyzing historical sales data, seasonal trends, social media sentiment, economic indicators, weather patterns, and consumer behavior trends. A Harvard Business Review study highlighted that retailers using unified commerce platforms report 23% higher profitability compared to those operating in technological silos.
Predictive inventory management not only improves operational efficiency but also enhances the customer experience. By ensuring product availability and tailoring assortments to match demand, retailers can avoid frustrating stockouts and provide shoppers with the items they want, when they want them.
Technology Ecosystem Driving Retail Innovation
The technological backbone of modern retail data science comprises multiple sophisticated components:
- Machine Learning Algorithms
- Predictive demand forecasting
- Customer segmentation
- Anomaly detection
- Personalized recommendation engines
- Cloud Computing Platforms
- Scalable data storage
- Real-time processing capabilities
- Flexible computational resources
- Secure data management
- Advanced Analytics Tools
- Sentiment analysis
- Predictive modeling
- Customer journey mapping
- Performance optimization
These technologies work in concert to transform raw data into actionable insights, enabling retailers to make data-driven decisions that optimize the entire customer experience.
Navigating Data Challenges
Despite the tremendous potential, retailers face significant challenges in implementing advanced data strategies:
- Data Integration Complexity
- Consolidating diverse data sources
- Ensuring data quality and consistency
- Managing disparate technological ecosystems
- Real-Time Processing Requirements
- Handling massive data volumes
- Implementing instant personalization
- Maintaining system responsiveness
- Privacy and Ethical Considerations
- Compliance with data protection regulations
- Transparent data usage policies
- Maintaining customer trust
Overcoming these challenges requires a strategic and holistic approach, using the right technology, governance frameworks, and organizational capabilities.
Emerging Trends in Retail Data Science
The future of retail data science promises even more transformative capabilities:
- Augmented reality shopping experiences
- Voice-activated personalized recommendations
- Predictive customer lifetime value modeling
- Autonomous inventory management systems
- Emotion-based marketing strategies
As technology continues to evolve, the most successful retailers will be those that can utilize the power of data science to create deeply personalized, seamless, and anticipatory shopping experiences.
Conclusion
Data science has fundamentally reimagined retail, transforming it from a transactional experience to a deeply personalized journey. As technological capabilities continue to expand, retailers who view data as a strategic asset, rather than merely an operational tool, will lead the marketplace.
The most successful organizations will be those that can turn every customer interaction into an opportunity for deeper connection, meaningful engagement, and sustainable growth.
Ready to revolutionize your retail strategy? To get started, retailers can reach out to Zenithive, who specialize in leveraging advanced analytics to revolutionize the customer experience.